Recommender Systems For Business
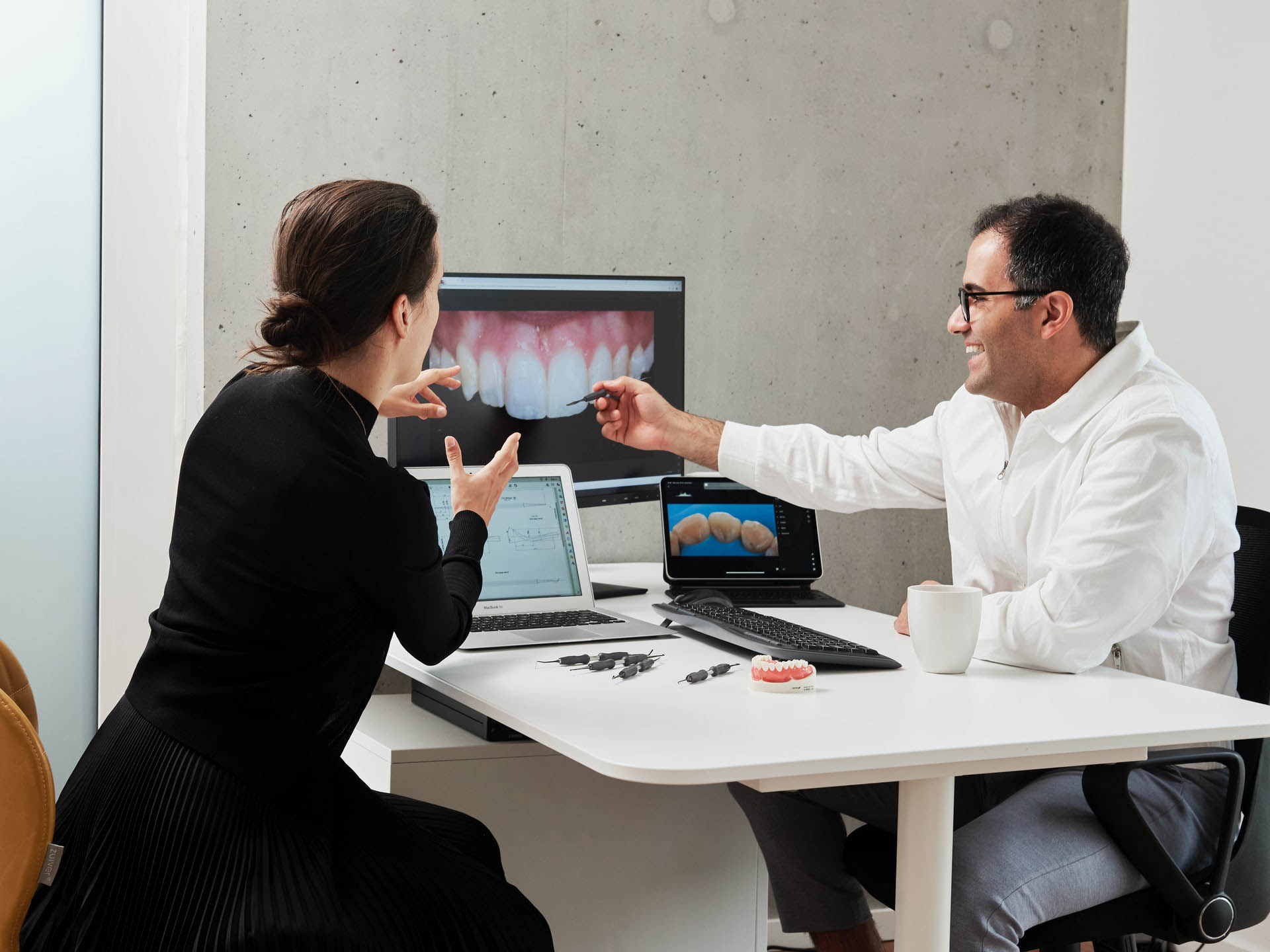
Recommender Systems For Business - A Gentle Introduction
With a majority of modern services and products now being offered predominately online, it can be hard to get to know your customers. Unlike running a local store where you get to know each person that comes in, online businesses can struggle to know exactly what their users are expecting.
So, how can your business use this, and what is the recommended steps to take?
Thanks to advances in machine learning, and deep learning specifically, it is now possible to get to know millions of customers completely online simply through their data. By using a data model to filter through your users' favorite products and interests, it is easier than ever to make recommendations to them for what they would enjoy to use or buy.
Read on to discover everything there is to know about recommender systems - and how can you use one to get to know your users and boost sales right now!
What is a recommender system?
If you have ever seen an advertisement on a web-page that seems strangely targeted to your hobbies, then you have probably already come across a recommender system without realizing it. Suggestions on Amazon and "What to Watch Next" on Netflix are all powered by recommender systems.
A recommender system, or recommendation data model, is essentially a type of machine learning model that filters throughout your previous interests and purchases and rates what you may be interested in. This ensures you only see products and information that you might truly be interested in and enjoy.
How does it work?
A recommendation system works in two different types of ways. Firstly, it will see what people who have similar tastes to you will like. This is done by gathering meta-data, such as whether they recommend a specific show on Netflix, and seeing what people tend to go on to watch after that show is over.
The other way a recommendation system will work is that it will assign a specific 'likelihood' rating to users and items they might like. It will then use filtering to remove any unlikely items or anomalies and only show you items linked to what you have already shown an interest in.
In more technical terms, a users' purchases or interests will be stored within a user and item matrix. Most ratings are either measured using a numerical system such as '1 out of 10' but some may use a binary rating. This is usually measured in a simpler way such as 'Clicked' or 'Watched'.
Most items in a User/Item matrix will be left blank, as this is what the machine is designed to fill in. These blanks will eventually be populated by recommended items or shows.
This matrix can then be compared with other users to find the 'nearest neighbor', or most similar user, and through deep learning, a machine can learn more about what users might like the most.
So, the next time you see a content-based recommendation or product recommendations, just know a complex machine learning model (commonly using the Singular Value Decomposition Method or clustering) is behind it.